Application cases
How to properly analyze hyperspectral images ?
Hyperspectral imaging has applications in many fields, including agriculture, environment, medicine and industry. This so-called “chemical” imaging makes it possible to characterize the chemical composition of products at each point of an image, thanks to the near-infrared spectrum measured for each pixel. It can thus be used to classify objects according to their composition, or to quantify compounds present on the surface and show their spatial distribution.
This scientific study presents the methodology for analyzing hyperspectral images in the laboratory, describing best practices in image acquisition, signal processing, image processing and finally chemometrics / Machine Learning methods applicable to hyperspectral images.
Images wee acquired with the SPECIM IQ (SPECIM – Konica Minolta) portable visible – near-infrared hyperspectral camera. The Machine LEarningmethods were developped on the PLS_Toolbox® and MIA_Toolbox® (Eigenvector Research Inc.).
Hyperspectral imaging has applications in many fields, including agriculture, environment, medicine and industry. This so-called “chemical” imaging makes it possible to characterize the chemical composition of products at each point of an image, thanks to the near-infrared spectrum measured for each pixel. It can thus be used to classify objects according to their composition, or to quantify compounds present on the surface and show their spatial distribution.
This scientific study presents the methodology for analyzing hyperspectral images in the laboratory, describing best practices in image acquisition, signal processing, image processing and finally chemometrics / Machine Learning methods applicable to hyperspectral images.
Images wee acquired with the SPECIM IQ (SPECIM – Konica Minolta) portable visible – near-infrared hyperspectral camera. The Machine Learning methods were developped on the PLS_Toolbox and MIA_Toolbox (Eigenvector Research Inc.).
Study carried out in collaboration with INRAE (UMR ITAP, Team COMIC) and IFV (IFV Occitanie – Languedoc Roussillon) as part of the VINIoT project, co-funded by the Interreg SUDOE program
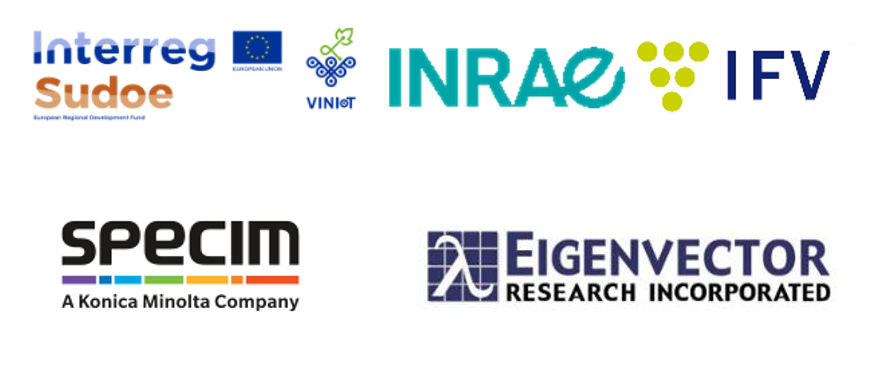
Support Vector Regression (SVR) on PLS Scores
applied to Near Infrared Data Sets
In NIR spectroscopy, the most common calibration method is the PLS regression. It is a linear multivariate calibration method, efficient on spectral data and easy to implement. However, this method reaches its limits when the data to predict is complex, for instance when many products or recipes are analyzed or when non-linear correlations are present.
In this study, conducted by Bruker Optics and Ondalys, two spectroscopic data sets were acquired with Bruker FT-NIR instruments MPA or TANGO. In each dataset, the heterogeneity of the products and the non-linearity between the parameters of interest and the spectra justified the use of advanced ML methods.
The Support Vector Machines (SVM) for quantitative analysis, called Support Vector Regression (SVR) were applied on PLS scores.
This simple and fast approach gave very satisfactory results, with lower errors with the SVR models on the PLS scores than the PLS models built with the optimal pretreatment and variable range selection. Moreover, SVR models were also built on the PLS scores without variable selection in order to reduce the optimization time.
In this case, the performances of the PLS models were strongly degraded whereas those of the SVR models remained satisfactory.
Study made with Bruker FT-NIR instruments MPA or TANGO
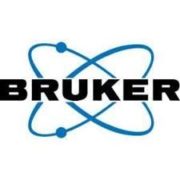
Comparison of Machine Learning Methods for Spectroscopic Data Analysis
The computing power of computers and volumes of data to be processed are increasing significantly. This makes Machine Learning (ML) more and more popular. Many methods exist and can be adapted to many fields. But what about Machine Learning for the spectroscopic data analysis?
To answer this question, several Machine Learning methods were compared on a spectroscopic data set (Near infrared Spectroscopy). “Classical” methods, such as PLS – Partial Least Squares Regression – et LWR – Locally Weighted Regression – are compared with 3 ML algorithms: SVM – Support Vector Machines – , ANN – Artificial Neural Networks and CART/RF – Classification and Regression Trees / Random Forest.
Data were acquired with a FOSS TECATOR InfratecTM spectrometer

Near-Infrared inter-spectrometer transfer
The B.I.P. – French interprofessional institute of the prune, wanted to develop a non-destructive decision aid-tool, applicable directly to the orchard in order to better estimate the maturity of the fruits and predict the optimal harvest date of the plums.
From large databases (more than 6000 samples) scanned on a laboratory spectrometer, the ASD LabSpec 4 (Malvern Panalytical), Ondalys has developed models for quantitative prediction of the sugar (° Brix) and acidity levels of plums in order to transfer the calibration on a micro-spectrometer MicroNIRTM (Viavi Solutions)
Following encouraging results, the study continues to transfer the models obtained to the portable MicroNIRTM spectrometer so that the fruit can be measured directly in the orchard.
Study funded by France AgriMer
Study made with a ASD LabSpec 4 spectrometer from Malvern Panalytical and a MicroNIRTM microspectrometer from VIAVI Solutions
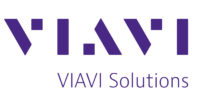
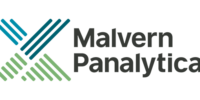
Application of MSPC (Multivariate Statistical Process Control) for industrial process supervision
Online process control has become essential in many industries. This monitoring improves the product quality and reduces costs, thanks to better production supervision and rapid intervention in case of drifts or anomalies.
In the case of continuous production, MSPC is an essential tool allowing to:
- monitor several criteria simultaneously, which can be simple criteria – temperature, pressure information, etc. – or complex ones – spectroscopic data, chromatograms, etc.
- take into account the interactions and the correlation structure existing between these different parameters.
The most important stage in the development of a MSPC model is defining the calibration set, i.e. identify the observations considered to be “normal”, when the system is stable. These observations, called NOC – Normal Operating Conditions, are used to build a PCA (Principal Component Analysis) model.
After choosing the relevant number of components, the test set observations are projected into the MSPC model. Thanks to the NOC determination and the statistical criteria, such as the Hotelling’s T² and the F-Residuals, the MSPC model makes it possible to identify process drifts or default occurrence.
The implementation of MSPC to monitor a manufacturing process of silicone polymers within Elkem company made it possible to successfully detect the different production phases – start, end, and possible breaks – moments during which the process is not stable. Lots which do not meet the product specifications in terms of quality criteria are also detected, allowing rapid corrections of the process.
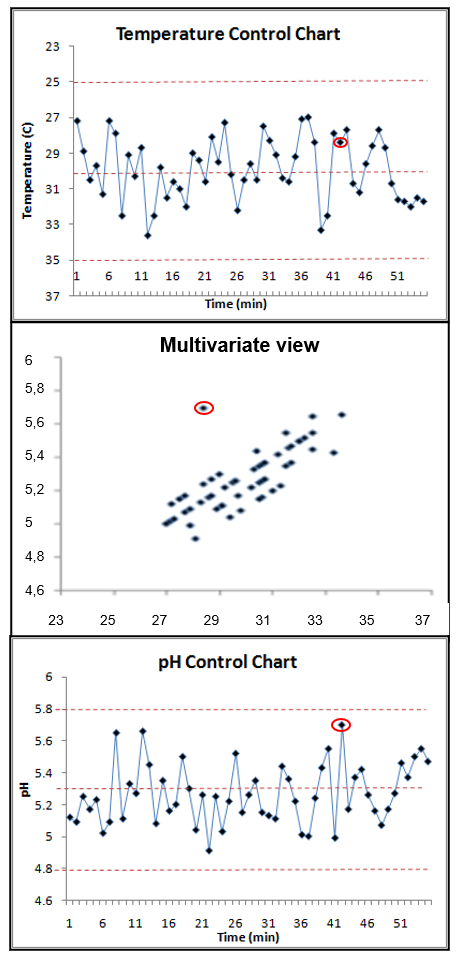
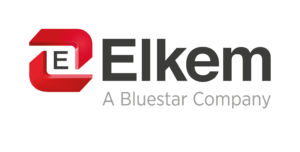
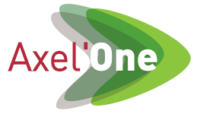
Study made with a Rxn4 spectrometer
from Kaiser Optical System Inc
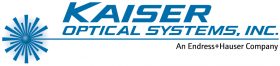
Comparison between SVM (Support Vector Machines) and PLS on spectral dataset
Support Vector Machines (SVM) are parts of the supervised Machine Learning methods. SVM were originally developed for classification objectives (pattern recognition), especially to discriminate between convex or hardly-separable classes. But, they are also very effective for quantitative prediction purposes.
This technique is very interesting to model non-linear relationships between the data or for intricate situations (e.g. complex parameter or concentrations close to the detection threshold).
In order to implement SVMs, 3 parameters must be optimized: a regularization parameter, the margin size and the non-linearity degree of the model, which is much simpler than training Artificial Neural Networks.
When predicting several quantitative parameters based on near-infrared spectroscopic data, SVM models provided significantly better results than PLS regression one (errors divided by 2).
This Machine Learning method brought a significant performance improvement vs PLS regression, due to the non-linearity present in the data sets (fat, protein and humidity contents in meat with NIR spectroscopy).
This project also demonstrated that with a rather small training set size, SVMs could show high performance and generalization power on an independent test set.
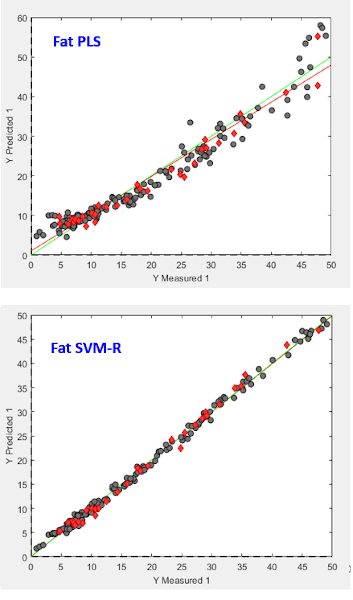
Study made with a TECATOR Infratec TM spectrometer from
FOSS

Model updating using the DOP orthogonalization method
In the frame of online monitoring of its polymerization processes, CERDATO, ARKEMA‘s research center, encountered problems in updating its spectroscopic calibrations. After several classical attempts, by adding new samples, their problem persisted.
They called Ondalys to train and support them in the use of different orthogonalization methods, in particular the Dynamic Orthogonal Projection (DOP) method.
Model corrected by DOP obtained better results than those developed with classical methods, while making it possible to diagnose problems that occurred on the production line.
Study made with a MATRIX-F spectrometer from
Bruker Optics
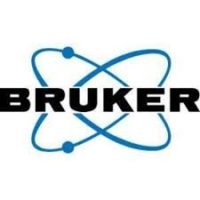
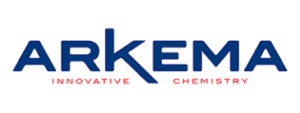
Characterization of powder blends by Near Infrared HyperSpectral Imaging (NIR-HIS)
Pharmaceutical industry faces daily issues of visual inspection of lyophilizate quality, fraud detection, or homogeneity evaluation of certain mixtures. In order to meet this need, HyperSpectral imaging (HIS) is interesting. This technique combines a camera and a spectrometer thus making it possible to simultaneously obtain spatial and spectral information on a given sample.
Therefeore, analysis using Near Infrared HyperSpectral Imaging (NIR-HIS) is very relevant to evaluate powder blends and their homogeneity. This kind of R&D study provides a better process understanding on the blending step. Thus, in the case of powder blending, optimal mixing times can be defined, with an end-process identification when the blend homogeneity is reached.
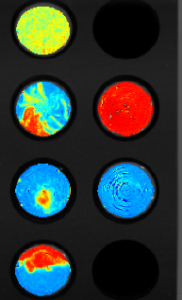
Study made with a HypeReal solution from
INDATECH Chauvin Arnoux
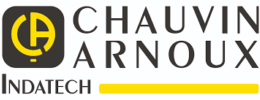
Prediction of the aromatic potential of grapes
The French institute of Wine and Vine (IFV) is always looking for improving the winemaking processes, from harvest to bottling process. The IFV often leads collaborative projects, in particular with Vinovalie, a group of wine coop from South -West of France.
One of their main problematic consists in identifying the aromatic potential of the grapes so as to direct the musts towards the most suitable winemaking process.
Aid-decision tools were developed during this project. They allow to define the optimized winemaking process for the grapes. They also make possible to increase the productivity and the wine quality. For the INES wine (AOP Fronton Rosé), the following results were obtained :
- + 10% productivity
- + 18% wines with high aromatic quality
- + 15% increase in price due to a higher aromatic quality
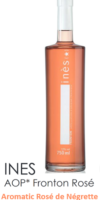
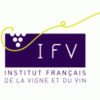

Study made with a WineScan TM spectrometer from
FOSS

Identification of Raw Materials using Near Infrared spectroscopy (NIR)
In order to gain time and efficiency for the product characterization, one of our customers wished to develop a method for identifying his Raw Materials (powders and liquids) using a Near infrared spectrometer.
This major player in the Pharmaceutical Industry, asked Ondalys to apply robust classification methods and develop robust identification models, that can be used systematically on incoming batches of Raw Materials.
Over 100 different Raw Materials (liquids and powders) are now characterized while arriving, thanks to near infrared spectroscopy and identification models developed by Ondalys.
Study made with a WineScan TM spectrometer from
FOSS

They talk about us
« We call for Ondalys for exploiting our data »
Our expertise to make sense of your data
With more than 15 years of experience in data analysis, chemometrics and machine learning, especially applicated to spectroscopic data, our team helps you in each step of your project.
You need a tailor-made training?
Our team study your request to offer you the most suitable and personalized training.